BLISful: Early lessons learned from the RED project
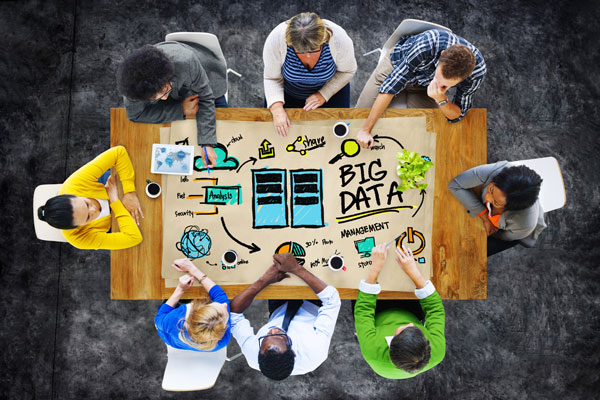
So far, the RED project has taught us a lot about team building, which, in the long run, may be among the more important lessons.

HEADLINE: The U.S. Announces the Creation of the Bureau of Leading Information Statistics
The U.S. federal government, together with tech giants Zillow, Willow, What’s-His-Nose-Book, Twitter, Pillow, Looped-In, Google, Billow, Amazon and others, has announced a public-private partnership to fund and create a federal agency to use “Big Data” to create and publish official information statistics. The private-public agency will create an information data statistics source that federal statistical agencies can use to better track the direction and dynamics of the economy and provide insights to how technology and information drives economic and social performance, as well as provide insights to evidence-based policy making.
The president applauded this, saying, “I think this is great. Just great. This is great because we, the country, need it.”
The agency, which will be referred to as BLIS, will serve as a pipeline for data from information and technology companies to the various federal statistical agencies. “There is a massive amount of data collected by the tech giants that can serve businesses, workers, researchers, policy makers … all the American people,” said a spokesperson from the U.S. government who spoke on the condition that we don’t call him out on his overuse of the term “going forward.”
“Going forward,” the official said, “big data companies and federal statistical personnel will collaboratively capture, refine and curate the data and information needed by the Census Bureau, the Bureau of Labor Statistics, the Bureau of Economic Analysis and other agencies.”
The spokesperson also mentioned that not all operational details have been hammered out. “Going forward,” he said, “we’ll need to ensure that BLIS data scientists don’t quit and go to work for one of the big tech companies, at least for several years. It would be awkward, to say the least, to have this federal agency serve as a training ground for workers to learn about the data and methods of one tech company, quit and apply that knowledge working for a competitor.”
The entire enterprise is rather tricky. There is a need to make the private companies comfortable with the arrangement and make the personnel and administrative burden for those firms as low as possible. “What’s in it for them,” the spokesperson rhetorically asked, “other than being good corporate citizens?”
“Enough!” The crowd shouted. “We can’t handle the truth … with fiction!”
Actually, all of the above is fiction. Except for the part that the need is great. The U.S. does need a public-private partnership with the imprimatur of an official federal bureau to refine and use big data for economic and social statistics.
Lesson 1: The need is great.
This is one of the lessons we have learned in the last few months working on the Regional Economic Development (RED) project.1 Big “unconventional” data that could be of great use for research, policy making, and monitoring the economy and the natural environment—among other applications—are difficult to get. There is a lot of information that goes untapped. Everyone across all institutional dimensions—private companies to federal agencies—needs to collaborate to bring unconventional economic data and statistics into the public domain that serves both private businesses and official federal statistics on economic and social dynamics.
There are people in government and in industry who are thinking about this. As it happens, there was a session at a conference on this very topic. There were also presentations on how researchers at companies like Google—economists no less—are using Google Trends data to nowcast some measures of economic performance and even develop a price index using price data scraped from web-based retailers.2 The impression one gets is that there is a lot of exciting work being done to apply unconventional data to many facets of social research,3 but it is fractured, siloed and very likely duplicative. Coordinating efforts and codifying standard practices would be in good order.
Having discussed this one lesson learned, what follows are three additional lessons learned from the RED project over the last few months.
Lesson 2: Data scientists and economists are strange bedfellows.
The second big lesson learned is that data scientists and economists are strange bedfellows. One might think that these two disciplines would fit hand in glove. Both are analytical, curious and want to figure things out. Both use high-powered, cutting-edge statistical methods. Despite the similarities, when data scientists and economists try to work together, there is something of a paradigm clash.
This clash was evident during the project’s second workshop. The first workshop was focused primarily on large conceptualizations and approaches (complex adaptive systems, for example), data science method and big questions. Regional science was not as dominant on the agenda. In the second workshop, the regional scientist/economists presented their research and methods to give the data scientists a better understanding of what they were about. What they are mostly about is applying theory and asking “why” questions. Economists spend a lot of effort trying to get to causality and to understand why. One needs to know why if one is doing policy-related work. (After all, the project subtitle carries the phase “precision policies.”)
The data scientists seemed content with correlation, descriptors and signals. While all very important and rich in providing insights, these do not enable theoretical development. There were a lot of questions, like, “What is your structural framework to analyze the (big) data? What are the expected results?” There was palpable discomfort in the room.
We left the room on good terms in the end, but one couldn’t help thinking that the “storming” phase of a team life cycle (see below) was going to be much longer than expected.
At the National Association for Business Economics (NABE) Tech Economics Conference: Economics in the Age of Algorithms, Experiments, and A.I. the following week, all the big tech firms were participating and sponsoring.4 Turns out that these big-data-creating firms not only hire data scientists and statisticians, but also economists. Google, for example, hired the preeminent economist Hal Varian as Chief Economist. It also turns out that when one puts the economists and the data scientists on the same team, it usually doesn’t go smoothly. Evidently, almost every day, big tech work teams suffer through the same data-science-versus-economics paradigm clash the RED project experienced the week before. A team is going to have a rough start if the members are not asking the same questions or don’t share the same expressed goal. (That said, the ultimate outcome may be a richer analysis, but the start may not be pretty.)Lesson 3: It helps to understand the life cycle of a team.
Which moves us to the third lesson learned. Implicit, if not almost explicit, in the discussion above is how teams perform over the course of a project. Back in the day, the life cycle for a team was made into a catchy quartet: forming, storming, norming and performing. The storming phase is the internal “Do I really have to work with these clowns?” and “Does the project manager have a clue?” Large, complex projects—like the RED project—have greater than average ambiguity, and thus more opportunities for misdirection and discontent.
The good news, however, is that it is liberating to know that this early experience in the storming phase is to be expected. It helps one deal with the early mess. We are not doomed. We simply dig in and hope we’ll get to norming soon.
Lesson 4: Working with unconventional data is hard.
The last lesson learned is that capturing and refining and curating and documenting and storing and retrieving unconventional data is hard. Very hard. While that hand-held device works like magic, the data the tech giants glean from our interactions on those devices and our computers does not work like magic. It takes considerable effort to make the data useful for any one particular purpose. The project has had to scale back (what some may consider manage expectations about) what data the project will be able to collect and eventually use to model and understand regional economic dynamics.
Conclusion
In closing, the assignment for this piece was to relate what the RED project has learned so far. No early empirical results to share to date. No new tools developed. We are still gathering data, most of which is conventional. The project team is defining the research questions that the unconventional data that we can collect will help answer. So far, we have learned more about team building, which, in the long run, may be among the more important lessons.
Notes
- For more about this project, see “IU researchers awarded $1.4 million grant to promote regional economic development,” News at IU, September 14, 2017, https://news.iu.edu/stories/2017/09/iu/releases/14-regional-economic-development.html and T. Slaper, “The Long View: New Data and Methods in Regional Economic Development,” Indiana Business Review, Winter 2017, www.ibrc.indiana.edu/ibr/2017/outlook/longview.html.
- The National Association for Business Economics (NABE) Tech Economics Conference occurred November 15-16 in Seattle, Washington: https://nabe.com/tec2017.
- E. L. Glaeser, H. Kim, and M. Luca, “Nowcasting the Local Economy: Using Yelp Data to Measure Economic Activity,” October 2017, https://papers.ssrn.com/sol3/papers.cfm?abstract_id=3037603.
- See note 2.